The fast affinity-based screening of DNA-encoded chemical libraries comprising billion of compounds allows to collect an enormous and unprecedented amount of physiochemical information. Artificial Intelligence and namely machine and neuronal learning hold the promise to effectively use this information, to rapidly obtain new insights into the structure– activity relationship for the design/synthesis of novel potent drug compound candidates as well as for guiding the assembly of second-generation lead-optimization libraries.
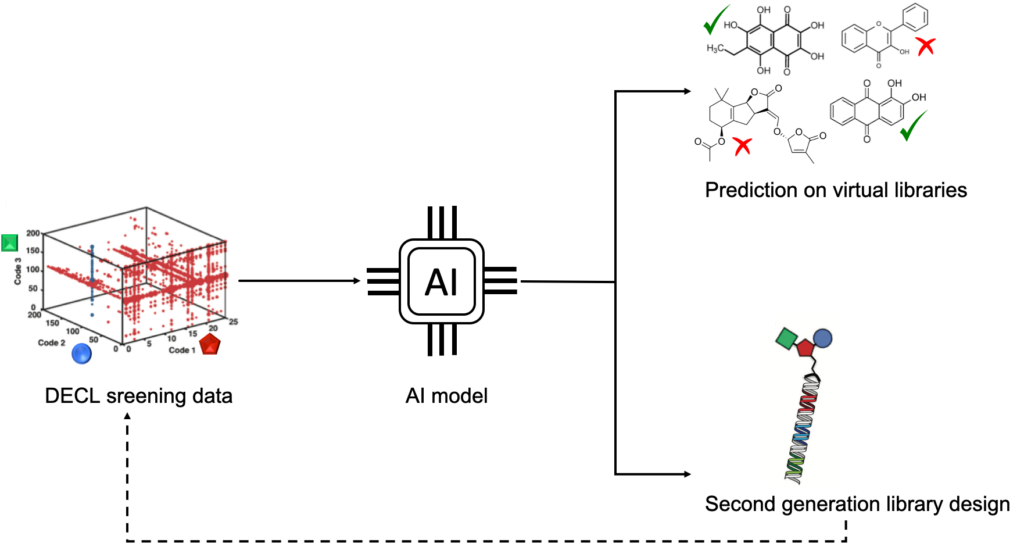
Figure 30: DECL selection information are used as training data for AI models. The trained models are then used to either predict hits from virtual libraries or to re-design second generation DECL libraries. Process can be then iterated.
Machine vs Neuronal learning
In 2020, Google Research Applied Science together with X-Chem reported the first use of the information gathered from the screening of a DNA-encode chemical library comprising about 108 unique compounds on three diverse target proteins (sEH, ERα and c-KIT associated protein) to train both a machine learning model (RF – Random Forest based model) as well as a graph convolutional neural network (GCNN), in order to in-silico screen a set of libraries (overall ∼88 million compounds) of easily synthesizable or inexpensive purchasable chemical compounds (e.g., Mcule database). On all the three test selection campaigns, GCNN model significantly outperformed the RF model in discovering more potent candidates. For c-KIT associated protein, the least productive protein in DECL screening, GCNN model was able to identify 78 hits with IC50 <30 μM.
Notably, the AI model developers were intentionally blinded to the names and nature of the targets at the time of model building: no known ligand data were given beyond the choice of the competitive inhibitors used in the selections (a parallel DECL selection were also performed in all cases including 40−100 μM of a suitable competitive inhibitor), and no explicit representation of the protein targets and 3D data were used in the AI priming. Eventually, only 2.2% (GCNN) and 3.0% (RF) of hit scaffolds for these targets were previously reported in bioactive molecule database (ChEMBL). This observation confirms that the newly discovered hits are also structurally novel.